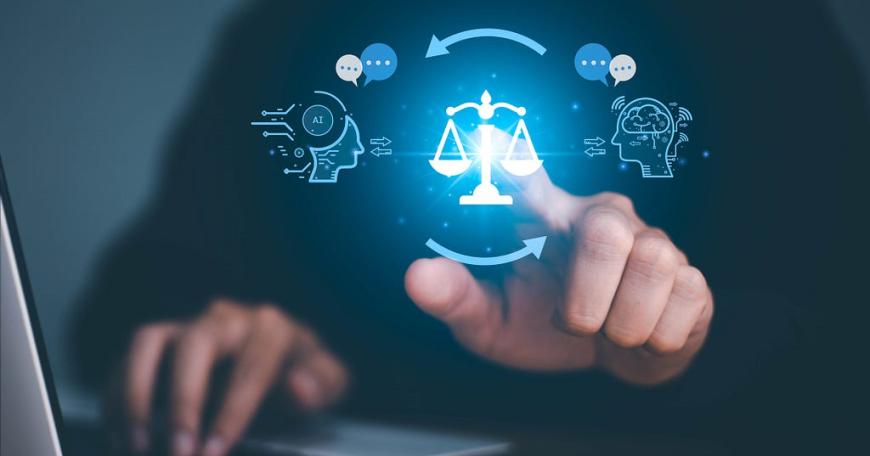
Ethics and AI-powered learning and assessment
By MIT Horizon
In the year or so since the public release of ChatGPT, learning has already being disrupted by generative AI. While potential benefits, such as AI-powered individual tutoring, are massive, there are risks, such as a decrease in social learning and important ethical considerations. While some of these issues pertain to a wide range of educational technologies, a number are unique to generative AI.
For example, many kinds of educational technologies follow learners to better understand their journeys and potentially offer individualized support. Struggling learners may be provided additional learning materials or offered more foundational content, while learners who are breezing through may be provided challenging problems or scenarios to practice. However, that the data behind these technologies can be used for other purposes should give us pause. Imagine that this data monitoring is happening on a corporate learning platform. Should the learner who goes through material faster be flagged to HR as a “better” candidate for promotion or receive other opportunities? Data that is really meant for one context, like performance in a learning platform, should not be repurposed in this way, particularly without clear communication to users about the various ways in which their data may be used.
AI and evaluation
It is likely that, in the near future, more scoring and assessment is going to be conducted by AI systems. On its own, this should not give us too much pause; there are already many computer-assisted ways of scoring performance that we are all used to, and no one today has any serious ethical concerns about using a Scantron sheet rather than requiring a human to score a multiple-choice problem. But what about more complex kinds of skills, such as writing or programming? What would need to be in place for AI to be able to score those in an ethically appropriate way? Here are a few principles to keep in mind for that scenario:
- Transparent: If the AI system can’t tell you why you have received a particular score, the system isn’t really providing a thorough evaluation. Most educators today will use a clear rubric to describe how a person is being graded, and then use that rubric to provide specific feedback regarding their performance on the required elements. Currently, most generative AI systems are black boxes, using complex neural networks to create something that is both appropriate but, fundamentally, randomly generated.
- Reproducible: Relatedly, for an evaluation to be fair, it must be reliable. Given the same system, the same input, and the same rubric, would the system provide identical scores and feedback? Given the ways in which generative AI, like ChatGPT, function, it is quite difficult to be assured that is the case.
- Bias-aware: AI systems are not free of bias, primarily due to the training data they are based on. For example, imagine you build a scoring system using essay responses on the topic of “My favorite sport” from high school students. Because of socioeconomic differences, you may end up finding that students who write about water polo tend to write more effectively. Rather than indicating that there is an important correlation between writing ability and water polo fandom, this likely indicates that only students from more advantaged backgrounds know anything about water polo, and those students also tend to have more academic support and resources that help them develop their writing skills. We wouldn’t want our system to end up giving extra points to anyone writing about water polo; we’d want to make sure that this kind of systemic bias is accounted for.
- Humans in the loop: People need to be prioritized and elevated above technologies. One way to do this is to provide learners the opportunity to provide feedback on their scores, and, if necessary, appeal the scoring decision. Over time, this kind of input will ultimately serve to make AI-powered systems more powerful, accurate, and trustworthy. In the near-term, it will help build trust and accountability in the evaluation process.
- Honesty and Integrity: Generative AI is also spurring a larger discussion on the idea of “cheating.” The truth is, lots of people are using generative AIs for things they are judged on, whether a homework assignment or a work project. In the learning context, this can lead to issues of fairness — students who do the work themselves and students who rely on help from generative AI may have different levels of mastery but end up with the same grades. But perhaps more critically, a student who uses ChatGPT to write for them won’t learn the material well or improve their ability to think and communicate about it, meaning they will not ultimately set themselves up for success. To address this challenge, many educators are exploring alternative assignments where learners use ChatGPT as part of their process but are ultimately responsible for something that goes beyond what the AI produces.
For example, one approach is to ask students to critique and improve on the writing that ChatGPT produces. This allows learners to shift their responsibility from “generating” (something that generative AI is built to do) to “creating quality,” something that humans are currently better equipped to do. Indeed, many writers are using generative AI tools in a similar way. Another approach is to create assignments that use specific instructor-created prompts to walk students through a particular kind of learning experience. An instructor could give all students a prompt that instructs the GenAI to act like a person who needs help understanding a certain concept and require the student to do the explaining. Students could then turn in their conversations with the GenAI and receive credit for the quality of their explanations.
AI and learning
Setting aside evaluation, there are many other important ethical considerations to keep in mind for AI and learning. Specifically, there are issues relating to who gets to use them and whether they are adequate as tools to help learning.
- Access and equity: As with many new technologies, equitable access is a concern. If only certain groups have access to beneficial tools, then existing disparities will widen and produce even more inequitable outcomes. Providers of learning tools need to consider their distribution models and whether there are ways to provide open-source or truly affordable license models that can benefit a wider range of learners. As different, more powerful versions of AI systems get released, cost can quickly become a deciding factor in the quality of learning experiences delivered.
- Content accuracy and appropriateness: One widely observed limitation of systems like ChatGPT is that they can produce false information (sometimes called hallucinations). For example, these systems may cite an article that doesn’t really exist or attribute some text to the wrong author. So, one important ethical consideration is ensuring the quality of the content that is generated. Obviously, having human experts review its work is an important fail-safe. Another approach could be to feed output from one large language model into other LLMs and generate a consensus view on its accuracy.
- Quality: While ChatGPT can be an amazing tool, it generally is not capable of producing something equal to expert human performance, particularly not without a lot of help in terms of customized prompts, tailored data inputs, and other more complex steps. In some contexts, providing access to something of average quality might be considered a big win; giving someone who doesn’t have access to any tutoring a ChatGPT-enabled AI tutor is likely going to help them immensely. But we should be careful to not elevate these tools too quickly, without evidence that they provide, at minimum, an equally impactful experience as other options. And, as discussed in our post on AI tutors, getting an AI to provide students with answers or feedback isn’t really “good enough.” Learning needs to be an active process, and tools that encourage the right kinds of learning activities will be much more effective than those that simply provide learners with information.
Generative AI is changing (really, has already changed) how we teach and learn, and it will only continue to drive disruptions in this space. It is up to those of us doing the teaching and learning to make sure that these changes are as fair, effective, and equitable as possible.
Originally published at https://horizon.mit.edu. Part of MIT Open Learning, MIT Horizon is comprised of a continuous learning library, events, and experiences designed to help organizations keep their workforce ahead of disruptive technologies.
Ethics and AI-powered learning and assessment was originally published in MIT Open Learning on Medium, where people are continuing the conversation by highlighting and responding to this story.